ABSTRACT
This chapter provides an overview of the disorders caused by iodine deficiency. Extensively referenced, it includes data on dietary sources of iodine, goitrogens, the effects of iodine deficiency throughout the lifecycle, the pathophysiology of iodine deficiency, as well as strategies for control and monitoring of the iodine deficiency disorders, such as iodized salt and iodized oil. It emphasizes the role of iodine deficiency in the development of brain damage and neurocognitive impairment, assessment of the iodine status of a population, the potential side effects of excessive iodine intake and current worldwide epidemiological data.
INTRODUCTION
This chapter provides a global overview of the disorders caused by iodine deficiency. Special emphasis will be put on recent developments such as the role of iodine deficiency in the development of brain damage and neurocognitive impairment, assessment of the iodine status of a population, strategies for control and monitoring of the iodine deficiency disorders (IDD), as well as side effects of iodine. Up to date information on IDD can be obtained by visiting the website of the Iodine Global Network (IGN) http://www.ign.org.
ETIOLOGY
DIETARY SOURCES OF IODINE
The native iodine content of most foods and beverages is low, and most commonly consumed foods provide 3 to 80 µg per serving (3-7). Major dietary sources of iodine in the USA, Europe and Australia are bread, milk and to a lesser extent seafood (3,4). Based on direct food analysis, mean intake of dietary iodine is ≈140 µg/day in Switzerland and 100-180 µg/day in Libya (3,6). Boiling, baking, and canning of foods containing iodised salt cause only small losses (of foods contain(8). Iodine content in foods is also influenced by iodine-containing compounds used in irrigation, fertilizers, and livestock feed. Iodophors, used for cleaning milk cans and teats in the dairy industry, can increase the native iodine content of dairy products through contamination of iodine containing residues (9); there are few data on the bioavailability of iodine or potential health risks from these iodophors. Traditionally, iodate was used in bread making as a dough conditioner, but it is being replaced by non-iodine-containing conditioners. Recommendations for daily iodine intake by age group are shown in Table 1.
Table 1: Recommendations for iodine intake (µg/day) by age or population group
Age or population groupa | U.S. Institute of Medicine (ref.5) | Age or population groupc | World Health Organization (ref.1) |
Infants 0–12 months b | 110-130 | Children 0-5 years | 90 |
Children 1-8 years | 90 | Children 6-12 years | 120 |
Children 9-13 years | 120 | ||
Adults n 9-13 yea | 150 | Adults >12 years | 150 |
Pregnancy | 220 | Pregnancy | 250 |
Lactation | 290 | Lactation | 250 |
a Recommended Daily Allowance. b Adequate Intake. c Recommended Nutrient Intake.
IODINE DEFICIENCY IN ANIMAL MODELS
Studies in rats have been carried out using the diet consumed by the people of Jixian village in China (10-13). This village was severely iodine deficient with 11% prevalence of endemic cretinism. The diet included available main crops (maize, wheat), vegetables, and water from the area with an iodine content of 4.5 µg/kg. After the dam had received the diet for 4 months, there was obvious neonatal goiter, fetal serum T4 was 3.6 µg/L compared to controls of 10.4 µg/L and they had higher 125I uptake and reduced brain weights. The density of brain cells was increased in the cerebral hemispheres. The cerebellum showed delayed disappearance of the external granular layer with reduced incorporation of 3H leucine in comparison to the control group.
Other more detailed studies have been carried out on the number and distribution of dendritic spines along the apical shaft of the pyramidal cells of the cerebral cortex of the rat (14). These dendritic spines can be accurately measured and have been studied in relation to both iodine deficiency and hypothyroidism. Their appearance and development reflects the formation of synaptic contacts with afferents from other neurons. In normal rats there is a progressive increase in the number of spines from 10 to 80 days of age.
These studies have demonstrated a significant effect of an iodine deficient diet on the number and distribution of the spines on the pyramidal cells of the visual cortex. This effect is similar to that of thyroidectomy. More detailed studies following thyroidectomy indicated the importance of the timing of the procedure. If carried out before the 10th day of life, recovery is unlikely to occur unless there is immediate replacement with L-T4. At 40 or 70 days, replacement can restore a normal distribution of spines even if there is a 30 day delay in its initiation. These differences confirm the need for early treatment of congenital hypothyroidism and prevention of iodine deficiency in the newborn infant in order to prevent brain damage and mental retardation.
Severe iodine deficiency has been produced in the marmoset (Callithrix Jacchus Jacchus) with a mixed diet of maize (60%), peas (15%), torula yeast (10%) and dried iodine deficient mutton (10%). The newborn iodine-deficient marmosets showed some sparsity of hair growth (15). The thyroid gland was enlarged with gross reduction in plasma T4 in both mothers and newborns, and was greater in the second pregnancy than in the first, suggesting a greater severity of iodine deficiency. There was a significant reduction in brain weight in the newborns from the second pregnancy, but not from the first. The findings were more striking in the cerebellum with reduction in weight and cell number evident and histological changes indicating impaired cell acquisition. These findings demonstrate the significant effects of iodine deficiency on the primate brain.
Severe iodine deficiency has been produced in sheep (16) with a low-iodine diet of crushed maize and pelleted pea pollard (8-15 ug iodine/kg) which provided 5-8 ug iodine per day for sheep weighing 40-50 kg. The iodine deficient fetuses at 140 days were grossly different in physical appearance in comparison to the control fetuses. There was reduced weight, absence of wool growth, goiter, varying degrees of subluxation of the foot joints, and deformation of the skull. (Fig. 2) There was also delayed bone maturation as indicated by delayed appearance of epiphyses in the limbs (17). Goiter was evident from 70 days in the iodine-deficient fetuses and thyroid histology revealed hyperplasia from 56 days gestation, associated with a reduction in fetal thyroid iodine content and reduced plasma T4 values. There was a lowered brain weight and DNA content as early as 70 days, indicating a reduction in cell number probably due to delayed neuroblast multiplication which normally occurs from 40-80 days in the sheep. Findings in the cerebellum were similar to those already described in marmoset (16).
A single intramuscular injection of iodized oil (1 ml = 480 mg iodine) given to the iodine deficient mother at 100 days gestation was followed by partial restoration of the lambs’ brain weight and body weight with restoration of maternal and fetal plasma T4 values to normal (16). Studies of the mechanisms involved revealed significant effects of fetal thyroidectomy in late gestation and a significant effect of maternal thyroidectomy on brain development mid gestation. The combination of maternal thyroidectomy (carried out 6 weeks before pregnancy) and fetal thyroidectomy produced more severe effects than that of iodine deficiency, and was associated with greater reduction in both maternal and fetal thyroid hormone levels (17). These findings in animal models confirm the importance of both maternal and fetal thyroid hormones in fetal brain development.
IODINE DEFICIENCY IN THE HUMAN LIFE CYCLE
The term IDD refers to all the ill-effects of iodine deficiency in a population that can be prevented by ensuring that the population has an adequate intake of iodine (1). These effects are listed in Table 2. Brain damage and irreversible mental retardation are the most important disorders induced by iodine deficiency: in 1990 it was estimated that among the 1572 million people in the world exposed to iodine deficiency (28.9 % of the then world population), 11.2 million were affected by overt cretinism, the most extreme form of mental retardation due to iodine deficiency and that another 43 million people were affected by some degree of itellectual impairment (18). Thus, iodine deficiency was a leading global cause of preventable mental impairment.
Table 2. The spectrum of iodine deficiency disorders, IDD (ref.1).
Fetus |
Miscarriage Stillbirths Congenital anomalies Increased perinatal morbidity and mortality Endemic cretinism |
Neonate |
Neonatal goiter Neonatal hypothyroidism Endemic neurocognitive impairment Increased susceptibility of the thyroid gland to nuclear radiation |
Child and adolescent |
Goiter (Subclinical) hypothyroidism Impaired mental function Retarded physical development Increased susceptibility of the thyroid gland to nuclear radiation |
Adult |
Goiter with its complications Hypothyroidism Impaired mental function Spontaneous hyperthyroidism in the elderly Iodine-induced hyperthyroidism Increased susceptibility of the thyroid gland to nuclear radiation |
Iodine Deficiency In Pregnancy
Iodine deficiency in the fetus is the result of iodine deficiency in the mother. The consequence of iodine deficiency during pregnancy is impaired synthesis of thyroid hormones by the mother and the fetus. An insufficient supply of thyroid hormones to the developing brain may result in neurocognitive impairment (19-25). The physiologic role of thyroid hormones is to ensure that normal growth and development occurs through specific effects on the rate of cell differentiation and gene expression. Thyroid hormone action is exerted through the binding of T3 to nuclear receptors which regulate the expression of specific genes in different brain regions during fetal and early postnatal life. The T3 which is bound to the nuclear receptors is primarily dependent on its local intracellular production from T4 via type II deiodinase and not from circulating T3.
Brain Development In Humans
Figure 1 shows the time course of the development of the brain and of thyroid function in the human fetus and neonate. Brain growth is characterized by two periods of maximal growth velocity (26). The first one occurs during the first and second trimesters between the third and the fifth months of gestation. This phase corresponds to neuronal multiplication, migration and organization. The second phase takes place from the third trimester onwards up to the second and third years postnatally. It corresponds to glial cell multiplication, migration and myelinization. The first phase occurs before fetal thyroid has reached its functional capacity. During this phase, the supply of thyroid hormones to the growing fetus is almost exclusively of maternal origin while during the second phase, the supply of thyroid hormones to the fetus is essentially, but not solely, of fetal origin (27).
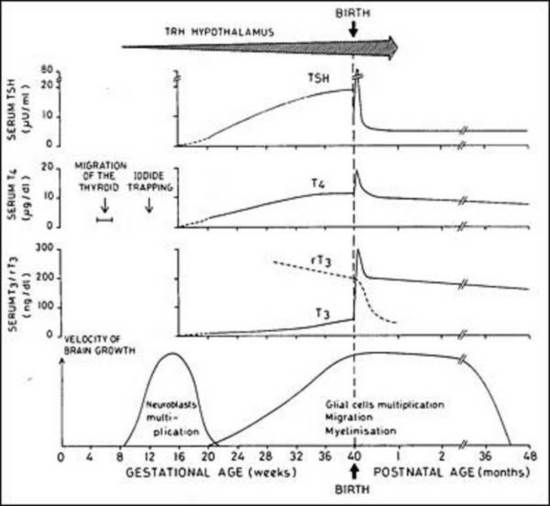
Figure 1. Ontogenesis of thyroid function and regulation in humans during fetal and early postnatal life in relation to the velocity of brain growth. From Delange and Fisher (ref.28).
In humans, T4 can be found in the first trimester coelomic fluid from 6 weeks of gestational age, long before the onset of secretion of T4 by the fetal thyroid, which occurs at the 24th week of gestation (29). Nuclear T3 receptors and the amount of T3 bound to these receptors increases six to tenfold between 10 and 16 weeks (30). The T4 and T3 found in early human fetuses up to mid gestation are likely to be entirely or mostly of maternal origin. As a consequence, infants born to women with hypothyroxinemia at 12 weeks gestation (fT4 concentrations; <10th percentile) had lower developmental scores (31). In addition, substantial amounts of T4 are transferred from mother to fetus during late gestation (32). The deiodinases are involved in the action of thyroid hormones in the brain, especially deiodinase D3 that is found in the uterine implantation site and in the placenta, producing rT3 from T4 and 3’,5’-T2 from T3 and thus having a protective effect to avoid an excess of thyroid hormone reaching the fetus.
Severe Iodine Deficiency in Pregnancy: Cretinism and Increased Fetal and Perinatal Mortality
The most serious adverse effect of iodine deficiency is damage to the fetus. Iodine treatment of pregnant women in areas of severe deficiency reduces fetal and perinatal mortality and improves motor and cognitive performance of the offspring. Severe iodine deficiency in utero causes a condition characterized by gross mental retardation along with varying degrees of short stature, deaf mutism, and spasticity that is termed cretinism. These disorders are described in detail below.
Mild-To-Moderate Deficiency in Pregnancy
The potential adverse effects of mild-to-moderate iodine deficiency during pregnancy in humans are unclear (33). Observational studies have shown associations between both mild maternal iodine deficiency and mild maternal thyroid hypofunction and decreased child cognition (33). In Europe, several randomized controlled trials of iodine supplementation in mild-to-moderately iodine deficient pregnant women have been done, and iodine reduced maternal and newborn thyroid size, and, in some, decreased maternal TSH; however, none of the trials showed an effect on maternal and newborn total or free thyroid hormone concentrations (33). No data are yet available from randomized placebo-controlled trials in regions of mild to moderate iodine insufficiency on the relation between maternal iodine supplementation and neurobehavioral development in the offspring (33).
Iodine Deficiency in the Neonate
An increased perinatal mortality due to iodine deficiency has been shown in Zaire from the results of a controlled trial of iodized oil injections alternating with a control injection given in the latter half of pregnancy (34). There was a substantial fall in infant mortality with improved birth weight following the iodized oil injection. Low birth weight of any cause is generally associated with a higher rate of congenital anomalies and higher risk through childhood. This has been demonstrated in the longer term follow up of the controlled trial in Papua New Guinea in children up to the age of 12 years (35) and in Indonesia (36).
A reduction of infant mortality has also been reported from China following iodine supplementation of irrigation water in areas of severe iodine deficiency. Iodine replacement has probably been an important factor in the national decrease in infant mortality in China (37).
Apart from mortality, the importance of thyroid function in the neonate relates to the fact that the brain of the human infant at birth has only reached about one third of its full size and continues to grow rapidly until the end of the second year (38). Thyroid hormone, dependent on an adequate supply of iodine, is essential for normal brain development as has been confirmed by the animal studies already cited.
Studies on iodine nutrition and neonatal thyroid function in Europe in the early 1980s confirmed the continuing presence of iodine deficiency affecting neonatal thyroid function and hence a threat to early brain development (39). A series of 1076 urine samples were collected from 16 centers from 10 different countries in Europe along with an additional series from Toronto, Canada and analyzed for their iodine content. The results of these determinations are shown in Table 3. The distribution was skewed so that arithmetic means were not used, but the results were expressed in percentiles. Some very high values were seen which could be attributed to the use of iodinated contrast media for radiological investigation of the mother during pregnancy. There was a marked difference in the results from the various cities. The high levels in Rotterdam, Helsinki and Stockholm differed from the low levels in Gottingen, Heidelberg, Freiburg and Jena by a factor of more than 10. Intermediate levels were seen in Catania, Zurich and Lille.
Table 3. Frequency distributions of urinary iodine concentrations in healthy term infants in 14 cities in Europe and in Toronto, Canada
Urinary Iodine Concentration (ug/L) | |||||
City | Number of infants | 10th Percentile | 50th Percentile | 90th Percentile |
Frequency (%) of values Below 50μg/L |
Toronto | 81 | 4.3 | 14.8 | 37.5 | 11.9 |
Rotterdam | 64 | 4.5 | 16.2 | 33.2 | 15.3 |
Helsinki | 39 | 4.8 | 11.2 | 31.8 | 12.8 |
Stockholm | 52 | 5.1 | 11.0 | 25.3 | 5.9 |
Catania | 14 | 2.2 | 7.1 | 11.0 | 38.4 |
Zurich | 62 | 2.6 | 6.2 | 12.9 | 34.4 |
Lille | 82 | 2.0 | 5.8 | 15.2 | 37.2 |
Brussels | 196 | 1.7 | 4.8 | 16.7 | 53.2 |
Rome | 114 | 1.5 | 4.7 | 13.8 | 53.5 |
Toulouse | 37 | 1.2 | 2.9 | 9.4 | 69.4 |
Berlin | 87 | 1.3 | 2.8 | 13.6 | 69.7 |
Gottingen | 81 | 0.9 | 1.5 | 4.7 | 91.3 |
Heidelberg | 39 | 1.1 | 1.3 | 4.0 | 89.8 |
Freiburg | 41 | 1.1 | 1.1 | 2.3 | 100.0 |
Jena | 54 | 0.4 | 0.8 | 2.2 | 100.0 |
Data on neonatal thyroid function was analyzed for four cities where enough newborns (30,000 - 102,000) had been tested. The incidence of permanent congenital hypothyroidism was very similar in the four cities but the rate of transient hypothyroidism was much greater in Freiburg, associated with the lowest level of urine iodine excretion, than in Stockholm, with intermediate findings from Rome and Brussels.
In developing countries with more severe iodine deficiency, observations have now been made using blood taken from the umbilical vein just after birth. Neonatal chemical hypothyroidism was defined by serum levels of T4 lesser than 3 ug/dl and TSH greater than 100 mIU/L). In the most severely iodine deficient environments in Northern India, where more than 50% of the population has urinary iodine levels below 25 ug per gram creatinine, the incidence of neonatal hypothyroidism was 75 to 115 per thousand births (40). By contrast in Delhi, where only mild iodine deficiency is present with low prevalence of goiter and no cretinism, the incidence drops to 6 per thousand. In control areas without goiter the level was only one per thousand.
There is similar evidence from neonatal observations in neonates in Zaire in Africa where a rate of 10% of biochemical hypothyroidism has been found (41). This hypothyroidism persists into infancy and childhood if the deficiency is not corrected, and results in retardation of physical and mental development (42). These observations indicate a much greater risk of mental impairment in severely iodine deficient populations than is indicated by the presence of cretinism.
Another important aspect of iodine deficiency in the neonate and child is an increased susceptibility of the thyroid gland to radioactive fall-out. Thyroidal uptake of radioiodine reached its maximum value in the earliest years of life and then declined progressively into adult life (43). The apparent thyroidal iodine turnover rate was much higher in young infants than in adults and decreased progressively with age. In order to provide the normal rate of T4 secretion, Delange (43) estimated the turnover rate for intrathyroidal iodine must be 25-30 times higher in young infants than in adolescents and adults. In iodine deficiency a further increase in turnover rate is required to maintain normal thyroid hormone levels. This is the reason for the greatly increased susceptibility of the neonate and fetus to iodine deficiency. Iodine deficiency also causes an increased uptake of the radioiodide resulting from nuclear radiation. Protection against this increased uptake can be provided by correction of iodine deficiency.
Iodine Deficiency in the Child
There is cross-sectional evidence that impairment of thyroid function evidenced in mothers and neonates in conditions of mild-to-moderate iodine deficiency affects the intellectual development of their offspring. Aghini-Lombardi et al. (44) reported that in children aged 6-10 years in an area in Tuscany who had mild iodine deficiency (64 μg iodine/day), the reaction time was delayed compared with matched controls from an iodine sufficient area (142 μg iodine/day). The cognitive abilities of the children were not affected. Similarly, it was reported that in an area of Southern Spain with mild iodine deficiency (median urinary iodine of 90 μg/L), the intelligence quotient (IQ) was significantly higher in children with urinary iodine levels above 100 μg/L (45).
A recent randomized controlled study in Albania in a moderately iodine deficient area showed that information processing, fine motor skill and visual problem solving significantly improved in school-children after iodine repletion of the population (46). In a randomized, placebo-controlled, trial in mildly-deficient New Zealand children aged 10-13 y, children were randomly assigned to receive a daily tablet containing either 150 μg iodine or placebo for 28 wk (47). At the end of the trial, the overall cognitive score of the iodine-supplemented group was 0.19 SDs higher than that of the placebo group (P<0.02). These controlled trials suggest that mild to moderate iodine deficiency could prevent children from attaining their full intellectual potential (46,47). As these anomalies were reversible, they probably result from lately acquired and reversible subclinical hypothyroidism, rather than from fetal and/or neonatal hypothyroidism.
In severe iodine deficiency, the frequency distribution of IQ in normal appearing children is shifted towards low values as compared to children who were not exposed to in utero iodine deficiency because of correction of the deficiency in the mothers before or during early gestation (48-49). In a meta-analysis of 19 studies on neuromotor and cognitive functions in conditions of moderate to severe iodine deficiency, Bleichrodt and Born (50) concluded that iodine deficiency resulted in a loss of 13.5 IQ points at the level of the global population.A more recent metanalysis conducted on studies in China produced a very similar result (51). Several of these studies are summarized in Table 4.
Table 4. Neurointellectual Deficits in Infants and Schoolchildren in Conditions of Mild to Moderate Iodine Deficiency
REGIONS | TESTS | FINDINGS | AUTHORS |
Spain |
Locally adpated BAYLEY McCARTHY CATTELL |
Lower psychomotor and mental development than controls | Bleichrodt et al. 1989 (52) |
Italy | |||
Sicily | BENDER- GESTALT |
Low preceptual integrative motor ability Neuromuscular and neurosensorial abnormalities |
Vermiglio et al. 1990 (53) |
Tuscany | WECHSLER RAVEN | Low verbal IQ, perception, motor and attentive functions | Fenzi et al. 1990 (54) |
Tuscany |
WISC Reaction time |
Lower velocity of motor response to visual stimuli |
Vitti et al. 1992 (55) Aghini-Lombardi et al. 1995 (44) |
India |
Verbal, pictorial learning tests Tests of motivation |
Lower capacities learning |
Tiwari et al. 1996 (56) |
Iran | Bender-Gestalt Raven | Retardation in psychomotor development | Azizi et al. 1993 (57) |
Malawi | Psychometric tests including verbal fluency | Loss of 10 IQ points as compared to iodine-supplemented controls | Shrestha 1994 (58) |
Benin | Battery of 8 non verbal tests exploring fluid intelligence and 2 psychomotor tests | Loss of 5 IQ points as compared to controls supplemented with iodine for one year | van den Briel et al. 2000 (59) |
Data from cross-sectional studies on iodine intake and child growth are mixed, with most studies finding modest positive correlations (60). In five Asian countries, household access to iodized salt was correlated with increased weight-for-age and mid-upper-arm circumference in infancy (61). However, controlled intervention studies of iodized oil alone and iodine given with other micronutrients have generally not found effects on child growth (60). In iodine-deficient children, impaired thyroid function and goiter are inversely correlated with IGF-1 and IGFBP-3 concentrations (62). Recent controlled trials found iodine repletion increased insulin-like growth factor (IGF)-1 and insulin-like growth factor binding protein (IGFBP)-3 and improved somatic growth in children (60).
Iodine deficiency in the adult
Iodine status is a key determinant of thyroid disorders in adults (63). Severe iodine deficiency causes goitre and hypothyroidism because, despite an increase in thyroid activity to maximise iodine uptake and recycling in this setting, iodine concentrations are still too low to enable sufficient production of thyroid hormone. In mild-to-moderate iodine deficiency, increased thyroid activity can compensate for low iodine intake and maintain euthyroidism in most individuals, but at a price: chronic thyroid stimulation results in an increase in the prevalence of toxic nodular goitre and hyperthyroidism in populations. Thus, a consequence of longstanding iodine deficiency in the adult (64-67) and child (68) is the development of hyperthyroidism, especially in multinodular goiters with autonomous nodules. The pathogenesis of this syndrome is discussed later in this chapter, in the section on side effects of iodine supplementation.
This high prevalence of nodular autonomy usually results in a further increase in the prevalence of hyperthyroidism if iodine intake is subsequently increased by salt iodisation. However, this increase is transient because iodine sufficiency normalises thyroid activity which, in the long term, reduces nodular autonomy. Increased iodine intake in an iodine-deficient population is associated with a small increase in the prevalence of subclinical hypothyroidism and thyroid autoimmunity; whether these increases are also transient is unclear. Thus, optimisation of population iodine intake is an important component of preventive health care to reduce the prevalence of thyroid disorders (63).
SPECIFIC IODINE DEFICIENCY DISORDERS
Endemic goiter
Epidemiology
Endemic goiter is characterized by enlargement of the thyroid gland in a significantly large fraction of a population group, and is generally considered to be due to insufficient iodine in the daily diet. Endemic goiter exists in a population when >5% of 6-12 year-old children have enlarged thyroid glands
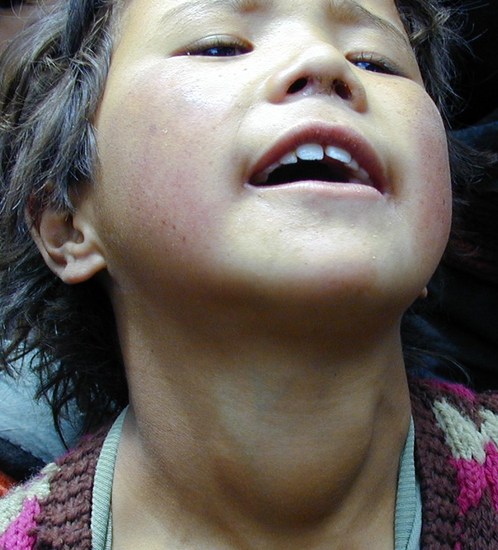
Figure 2 shows a young girl with a soft diffuse goitre and an elderly woman with a huge, longstanding multinodular goiter, both resulting from iodine deficiency.
Most mountainous districts in the world have been or still are endemic goiter regions. The disease may be seen throughout the Andes, in the whole sweep of the Himalayas, in the European Alps where iodide prophylaxis has not yet reached the entire population, in Greece and the Middle Eastern countries, in many foci in the People's Republic of China, and in the highlands of New Guinea. There are or were also important endemias in non-mountainous regions, as for example, the belt extending from the Cameroon grasslands across northern Zaire and the Central African Republic to the borders of Uganda and Rwanda, as well as in Holland, Central Europe and the interior of Brazil. An endemic existed in the Great Lakes region in North America until it was corrected by iodized salt in the early 1900s.
Goiter maps of various countries have been repeatedly drawn, requiring modification as successful prophylactic measures have been introduced. Although goiter was an important problem in many regions of the United States in the past (69), more recent US surveys have shown it in no more than 4-11% of schoolchildren, with evidence of continued adequate iodine nutrition in the country since 1988 (70,71). This finding is a testimony to the effectiveness of iodine prophylaxis in preventing endemic goiter. The world or regional distribution of goiter was exhaustively reviewed by Kelly and Snedden in 1960 (72) and, most recently, in 2005 (73).
These surveys reveal striking differences in the rate of goiter in different endemic regions and even in adjacent districts. The geographic unevenness of an endemia undoubtedly has much to do with the habits of the population and their ability and/or desire to import iodine containing foods. In attempting to account for the variability in the expression of endemic goiter from one locality to the next, the availability of iodine should be investigated before searching for some other subtle dietary or genetic factors. The key to the problem almost always lies in the availability of iodine. One must also consider the possibility that an observed goiter rate may not reflect current conditions, but rather may be a legacy of pre-existing iodine deficiency that has not yet been entirely resolved by an improvement in the supply of iodine. The assessment of goiter in a population, and its limitations, are discussed in the section on assessment of the IDD status of the population.
Etiology
Iodine Deficiency
The arguments supporting iodine deficiency as the cause of endemic goiter are four: (1) the close association between a low iodine content in food and water and the appearance of the disease in the population; (2) the sharp reduction in incidence when iodine is added to the diet; (3) the demonstration that the metabolism of iodine by patients with endemic goiter fits the pattern that would be expected from iodine deficiency and is reversed by iodine repletion; and 4) iodine deficiency causes changes in the thyroid glands of animals that are similar to those seen in humans (74,75). Almost invariably, careful assessment of the iodine intake of a goitrous population reveals levels considerably below normal.
Goitrogenic factors
Although the relation of iodine deficiency to endemic goiter is well established, other factors may be involved. A whole variety of naturally occurring agents have been identified that might be goitrogenic in man (76,77). It should be recognized that goitrogens are usually active only if iodine supply is limited and/or goitrogen intake is of long duration. Many of these have only been tested in animals and/or have been shown to possess antithyroid effects in vitro. These compounds belong to the following chemical groups:
- Sulfurated organics (like thiocyanate, isothiocyanate, goitrin and disulphides)
- Flavonoids (polyphenols)
- Polyhydroxyphenols and phenol derivatives
- Pyridines, phthalate esters and metabolites,
- Polychlorinated (PCB) and polybrominated (PBB) biphenyls
- Organochlorines (like DDT)
- Polycyclic aromatic hydrocarbons (PAH)
- Inorganic iodine (in excess)
Gaitan (76) divides goitrogens into agents acting directly on the thyroid gland and those causing goiter by indirect action. The former group is subdivided into those inhibiting transport of iodide into the thyroid (like thiocyanate and isothiocyanate), those acting on the intrathyroidal oxidation and organic binding process of iodide and/or the coupling reaction (like phenolic compounds) some phthalate derivatives (disulfides and goitrin) and those interfering with proteolysis, dehalogenation and hormone release (like iodide and lithium).
Indirect goitrogens increase the rate of thyroid hormone metabolism (like 2,4-dinitrophenol, PCB's and PBB's). Soybean, an important protein source in many third world countries, interrupts the enterohepatic cycle of thyroid hormone (78) and may cause goiter when iodine intake is limited.
Some of these goitrogens are synthetic and are used medicinally. Others occur in certain widely used food plants (79). The initial recognition of dietary goitrogenesis is attributed to Chesney et al. (80) who in 1928 found that rabbits fed largely on cabbage developed goiters. In 1936, Barker (81) found that thiocyanate used in large doses to treat hypertension resulted in goiter. In 1936, Hercus and Purves (82) reported their studies on the production of goiter in rats by feeding the seeds of several species of Brassica (rape, choumoellier, turnip, etc.). Both Mackenzie and MacKenzie (83) and Astwood (84) found in the 1940’s that certain drugs such as thiourea and related compounds caused hyperplasia of the thyroid when administered to rats. Their investigations quickly led to the introduction of the thionamide series of antithyroid drugs, now so familiar in clinical therapeutics.
Thiocyanate and precursors of thiocyanate, such as the cyanogenic glycosides, form another group of widely distributed natural antithyroid substances. They have been found particularly in the widely used tuber cassava (manioc) (85). Cassava causes goiter when fed to rats (86). Certain sulfur-containing onion volatiles are also goitrogenic (87). All of these substances interfere with the accumulation of thyroidal iodide, an effect that usually can be overcome by an increasing iodine intake.
Delange et al. (88) observed a striking difference in incidence of goiter in two regions of an isolated island in the Kivu Lake in Eastern Dem. Rep. of Congo, although the iodine intake of both groups was approximately the same. There was a major difference in the use of cassava. Cassava has been implicated as a contributing factor in endemic goiter in Zaire (89,90). In a study of several communities in the Ubangi region of Zaire, a relationship between goiter, thiocyanate and iodide excretion was described. The thiocyanate was derived from intestinal breakdown of the cyanogenic glycoside, linamarin, from cassava and its conversion to thiocyanate by the liver. The results indicated a reciprocal relationship between iodide and thiocyanate in that increasing amounts of iodide protected increasingly against the thiocyanate derived from the cassava (89). Thiocyanate may cross the human placenta (89, 91) and affect the thyroid of the fetus.
Excessive intake of iodine may cause goiter. A localized endemia has been reported on the coast of Hokkaido in Northern Japan (92). In this district the diet contained a huge amount of seaweed, and excretion of 127I in the urine exceeded 20 mg/day. The uptake of RAI by the thyroid was low, and some of it could be discharged by administration of thiocyanate, indicating impairment of organification. Similar findings have been reported from coastal (93) and continental (94) China.
Firm evidence for goitrogenic action in humans has only been shown for a few compounds: thiocyanate, goitrin, resorcinol, dinitrophenol, PBB's and its oxides, excess iodine and high doses of lithium (77). A definite role in endemic goiter has only been proved for thiocyanate and sulfurated organics, although substantial and circumstantial evidence favors the view that natural goitrogens, acting in concert with iodine deficiency, may determine the pattern and severity of the condition. An example is the possible role of the consumption of pearl millet in the etiology of endemic goiter in Sudan (95).
Selenium deficiency may have profound effects on thyroid hormone metabolism and possibly also on the thyroid gland itself (96-98). In this situation the function of type I deiodinase (a selenoprotein) is impaired. Type I deiodinase plays a major role in T4 deiodination in peripheral tissues. It has been shown that when, in an area of combined iodine and selenium deficiency, only selenium is supplemented, serum T4 decreases (99). This effect is explained by restoration of type I deiodinase activity leading to normalization of T4 deiodination while T4 synthesis remains impaired because of continued iodine deficiency.
Selenium deficiency also leads to a reduction of the selenium containing enzyme glutathione peroxidase. Glutathione peroxidase detoxifies H2O2 which is abundantly present in the thyroid gland as a substrate for the thyroperoxidase that catalyzes iodide oxidation and binding to thyroglobulin, and the oxidative coupling of iodotyrosines into iodothyronines. Reduced detoxification of H2O2 may lead to thyroid cell death (96,100). Elevated H2O2 levels in thyrocytes may be more toxic under situations of increased TSH stimulation such as is present in areas with severe iodine deficiency. Extensive epidemiological data collected in China indicated that all selenium-deficient areas were IDD-endemic areas. However, the reverse is not true: IDD can be very severe in many selenium-rich areas (101).
Deficiencies of iron (102,103) and vitamin A (104) may also have a goitrogenic effect in areas of iodine deficiency (Table 5).
Table 5. Dietary Goitrogens
Goitrogen | Mechanism |
Foods | |
Cassava, lima beans, linseed, sorghum, sweet potato | Contain cyanogenic glucosides; they are metabolized to thiocyanates that compete with iodine for thyroidal uptake |
Cruciferous vegetables: cabbage, kale, cauliflower, broccoli, turnips, rapeseed | Contains glucosinolates; metabolites compete with iodine for thyroidal uptake |
Soy, millet | Flavonoids impair thyroid peroxidase activity |
Nutrients | |
Selenium deficiency | Accumulated peroxides may damage the thyroid, and deiodinase deficiency impairs thyroid hormone synthesis |
Iron deficiency | Reduces heme-dependent thyroperoxidase activity in the thyroid and may blunt the efficacy of iodine prophylaxis |
Vitamin A deficiency | Increases TSH stimulation and goiter through decreased vitamin A-mediated suppression of the pituitary TSHβ gene |
Pathology
There are no gross or microscopic features that distinguish the thyroid of endemic goiter from changes that may appear in simple and sporadic goiter. The changes evolve through stages. In the very young, or in older patients who have lived under constant iodide deprivation, the finding is extreme hyperplasia. In some instances only a cellular organ is found, with little or no colloid. (Figure 3) The evolution of pathologic findings in humans have been detailed and well illustrated by Correa (105) and Studer and Ramelli (106) and follow the pattern of events first described by Marine (107) and known as the Marine cycle. In this formulation, repeated episodes of hyperplasia induced by iodine deficiency are followed by involution and atrophy, the result being a gland containing a mixed bag of nodules, zones of hyperplasia, and involuting, degenerative, and repair elements.
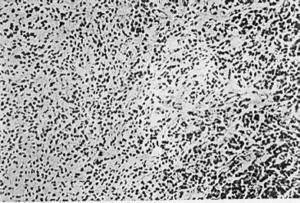
Figure 3. Histological section of large goiter removed because of pressure symptoms in Papua New Guinea, showing intense hyperplasia with no colloid. From Buttfield and Hetzel (140).
Diagnosis
A diagnosis of endemic goiter implies that the cause is known, or at least strongly suspected. Usually water and food are found to have very low iodine content. The thyroid glands are often diffusely enlarged in childhood, but are almost always nodular in adults. The typical laboratory findings are elevated radioiodine thyroidal uptake (RAIU), normal or low T4 and FT4 levels, normal or elevated T3 levels, normal or elevated TSH levels, and diminished urinary 127I excretion. RAIU is typically suppressible when thyroid hormone is given, but not always. Scanning with radioiodine or TcO4- shows a mottled distribution of the isotope. Antithyroglobulin or thyroperoxidase antibodies are usually absent. In an area of endemic goiter, the diagnosis can be presumed if the goiter is a community problem, but one must always be wary of missing individual patients with thyroiditis, thyrotoxicosis or thyroid carcinoma.
Pathophysiology
When iodine intake is abnormally low, adequate secretion of thyroid hormones may still be achieved by marked modifications of thyroid activity. These adaptive processes include stimulation of the trapping mechanism of iodide by the thyroid as well as stimulation of the subsequent steps of the intrathyroidal metabolism of iodine leading to preferential synthesis and secretion of T3. They are triggered and maintained by increased secretion of TSH. The morphological consequence of prolonged thyrotropic stimulation is thyroid hyperplasia (108).
The first functional consequence of iodine deficiency is an increase in the uptake of iodide by the thyroid mediated via a transmembrane protein, the sodium iodide symporter (NIS) (109). There is a clear inverse relation between iodine supply and thyroidal uptake of radioiodide. The increased uptake may be accompanied by and may result from an increase in the serum levels of TSH. However, elevated TSH in endemic goiter is usually systematically found only in conditions of extreme iodine deficiency. In conditions of mild iodine deficiency, elevated TSH is typically found in only a small fraction of subjects, usually the youngest (110). It is possible that it is the sensitivity of the thyroid to TSH rather than the TSH level itself that mainly varies with iodide supply. However, whatever the relative roles of TSH levels and sensitivity to TSH, the thyroid is stimulated as demonstrated by increased secretion and elevated serum levels of thyroglobulin.
For any adequate adjustment of iodine supply to the thyroid, iodide trapping must fulfill two conditions. First, it must reduce the amount of iodide excreted in the urine to a level corresponding to the level of iodine intake in order to preserve the preexisting iodine stores. Second, it must ensure the accumulation in the thyroid of definite amounts of iodide per day, estimated at least 100 μg/day in adolescents and adults. The increase in the iodide clearance by the thyroid despite the decrease in the serum concentration of iodide maintains a normal absolute uptake of iodide by the thyroid and an organic iodine content of the thyroid which remains within the limits of normal (i.e., 10-20 mg) as long as the iodine intake remains above a threshold of about 50 μg/day. Below this critical level of iodine intake, despite a further increase of thyroid iodide clearance, the absolute uptake of iodide diminishes and the iodine content of the thyroid decreases with functional consequences resulting in the development of a goiter (111,112).
Thyroid hyperplasia induced by iodine deficiency is associated with an altered pattern of thyroid hormonogenesis: the abnormal configuration of the poorly iodinated thyroglobulin in the thyroid colloid is accompanied by an increase in poorly iodinated compounds, monoiodotyrosine (MIT) and T3, and a decrease in diiodotyrosine (DIT) and T4. The increase of the MIT/DIT and T3/T4 ratios is closely related to the degree of iodine depletion of the gland (113).
The T3/T4 ratio in the serum may be elevated in conditions of iodine deficiency because: 1) thyroidal secretion of T4 and T3 is in the proportion in which they exist within the gland; and/or 2) preferential secretion of T3 or increased peripheral conversion of T4 to T3. The shift to increased T3 secretion plays an important role in the adaptation to iodine deficiency because T3 possesses about 4 times the metabolic potency of T4 but requires only 75 % as much iodine for synthesis.
However, efficient adaptation to iodine deficiency is possible in the absence of goiter as demonstrated in nongoitrous patients in endemic goiter areas such as New Guinea (114) and the Congo (115). Moreover, adequate adaptation to iodine deficiency has been demonstrated in areas of severe iodine deficiency in the absence of endemic goiter (116). This clearly indicates that goiter is not required for achieving efficient adaptation to iodine deficiency. Rather, in these conditions, efficient adaptation to iodine deficiency is possible thanks to a high iodide trapping capacity but with only a slight enlargement of the thyroid. At this stage, the characteristic hyperplastic picture includes abundant parenchyma, high follicular epithelium and rare colloid.
On the contrary, in large goiters, the major part of the gland is occupied by extremely distended vesicles filled with colloid with a flattened epithelium. The mechanism responsible for the development of colloid goiter is not fully understood (117), but it does not appear to be TSH hyperstimulation. It must be the consequence of an imbalance between thyroglobulin synthesis and hydrolysis, i.e. secretion. In these conditions, iodide is diluted while thyroglobulin is in excess, resulting in a lesser degree of iodization of thyroglobulin and, consequently, in a decrease in iodothyronine synthesis and secretion (118). Hydrolysis of large amounts of poorly iodinated thyroglobulin will result in an important leak of iodide by the thyroid and enhanced urinary loss of iodide, further aggravating the state of iodine deficiency (119). Therefore, large colloid goiters in endemic iodine deficiency represent maladaptation instead of adaptation to iodine deficiency because they may produce a vicious cycle of iodine loss and defective thyroid hormones synthesis.
Endemic cretinism
Epidemiology
When McCarrison described cretinism in north-western India during the first decade of this century (120), he delineated a neurologic form, with predominantly neuromotor defects, including strabismus, deaf-mutism, spastic diplegia, and other disorders of gait and coordination. The patients usually had a goiter. The other form, which he called the myxedematous form, showed evidence of severe hypothyroidism, short stature, and markedly delayed bone and sexual maturation. The patients usually had a thyroid normal in size and position, and were seldom deaf.
Neurological Cretinism
The three characteristic features of neurological endemic cretinism in its fully developed form are extremely severe mental deficiency together with squint, deaf mutism and motor spasticity with disorders of the arms and legs of a characteristic nature. (Figure 4). As would be expected with a deficiency disease, there is a wide range in the severity of the clinical features in the population affected (120-122).
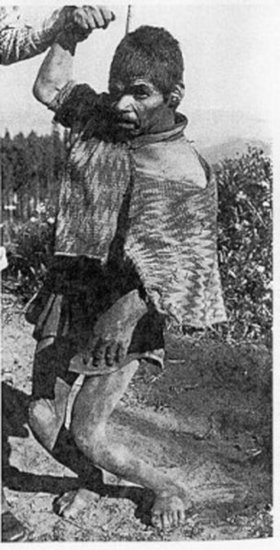
Figure 4 (a). Male from Ecuador about 40 years old, deaf-mute, unable to stand or walk. Use of the hands was strikingly spared, despite proximal upper-extremity spasticity.
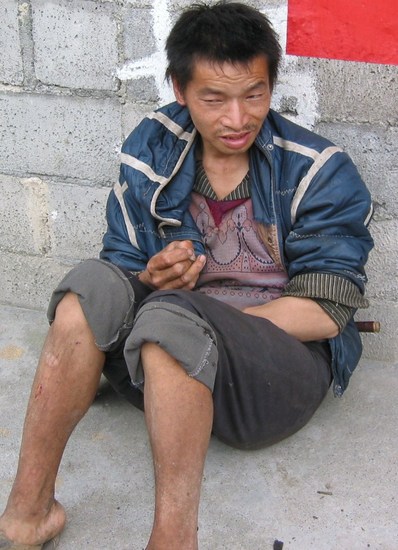
Figure 4 (b). Male from South western China with the typical facies of neurological cretinism, who is also deaf-mute and suffering from less severe proximal muscle weakness in lower limbs.
Mental deficiency is characterized by a marked impairment of the capacity for abstract thought but vision is unaffected. Autonomic, vegetative, personal, social functions and memory appear to be relatively well preserved except in the most severe cases.
Deafness is the striking feature. This may be complete in as many as 50% cretins. It has been confirmed by auditory brain stem evoked potential studies which showed no cochlear or brain stem responses even at the highest sound frequencies. These findings suggest a cochlear lesion. In subjects with reduced hearing a high tone defect is apparent. Deafness is sometimes absent in subjects with other signs of cretinism. Nearly all totally deaf cretins are mute and many with some hearing have no intelligible speech.
The motor disorder shows a characteristic proximal rigidity of both lower and upper extremities and the trunk. There is a corresponding proximal spasticity with markedly exaggerated deep tendon reflexes at the knees, adductors and biceps. Spastic involvement of the feet and hands is unusual or, if present, is much milder than that of the proximal limbs. Function of the hands and feet is characteristically preserved so that most cretins can walk. This observation is very useful in differentiating cretinism from other forms of cerebral palsy commonly encountered in endemic areas, such as cerebral palsy from birth injury or meningitis.
In addition to frank cretinism, a larger proportion of the population suffers from some degree of intellectual impairment and coordination defect. Comparative population based neuropsychological assessments of children in areas of iodine deficiency compared with areas with adequate iodine intake confirm a shift of the intelligence curve to the left in the iodine deficient areas. Careful examination of affected individuals in such areas reveals a pattern of neurological involvement similar to that seen in frank cretins, although of milder degree. In assessing these less severe defects, nonverbal tests are most helpful and school progress is a good indicator. After the age of 3 years drawings are very useful, indicating a defect in visual motor integration. Finally, elevated hearing thresholds have been reported in children with no other signs of endemic cretinism in conditions of mild iodine deficiency (123).
DeLong (124) suggests that the neuropathological basis of the clinical picture includes underdevelopment of the cochlea for deafness; maldevelopment of the cerebral neocortex for mental retardation; and maldevelopment of the corpus striatum (especially putamen and globus pallidus) for the motor disorder. The cerebellum, hypothalamus, visual system, and hippocampus are relatively spared.
Pathophysiology of neurological cretinism
Developmental neuropathology and available epidemiologic data suggest that the period from about 12-14 weeks until 20-30 weeks of gestation may be the critical period during which damage occurs (19). Cortical and striatal neuron proliferation, migration, and early formation of neuropil occur between 12 and 18 weeks. Cochlear development occurs at the same time. These data correlate well with the data from the Papua New Guinea trial which indicated that iodine repletion must occur by three months of pregnancy to prevent cretinism (35).
Studies already cited above on the effect of iodine deficiency on brain cell development in the newborn rat, sheep and marmoset suggest that iodine deficiency has an early effect on neuroblast multiplication. Brain weight is reduced and there are a reduced number of cells, a greater density of cells in the cerebral cortex and reduced cell acquisition in the cerebellum. Because maternal thyroxine crosses the placenta, it is now envisaged that neurological cretinism is predominantly caused by maternal hypothyroidism due to iodine deficiency (125). It has been suggested that an autosomal recessive predisposition, besides maternal iodine deficiency, may play an etiological role in neurological cretinism (126).
Myxedematous Cretinism
The typical myxedematous cretin (Fig 5) has a less severe degree of mental retardation than the neurological cretin, but has all the features of extremely severe hypothyroidism present since early life, as in untreated sporadic congenital hypothyroidism (127-129): severe growth retardation, incomplete maturation of the facial features including the naso-orbital configuration, atrophy of the mandibles, puffy features, myxedematous, thickened and dry skin, dry and decreased hair, eyelashes and eyebrows and much delayed sexual maturation.
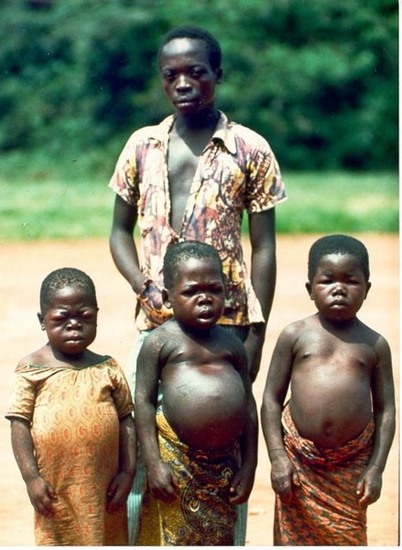
Figure 5. Myxedematous endemic cretinism in the Democratic Republic of Congo. Four inhabitants aged 15-20 years : a normal male and three females with severe longstanding hypothyroidism with dwarfism, retarded sexual development, puffy features, dry skin and hair and severe mental retardation.
Contrasting with the general population and with neurological cretinism, goiter is usually absent and the thyroid is often not palpable, suggesting thyroid atrophy. This diagnosis is confirmed by thyroid scans that show a thyroid in the normal location but of small volume with a very heterogeneous and patchy distribution of the tracer (114). Thyroidal uptake of radioiodine is much lower than in the general population. The serum levels of T4 and T3 are extremely low, often undetectable, and TSH is dramatically high. Markedly enlarged sella turcicas have been demonstrated, suggesting pituitary adenomas (130).
Myxedematous cretinism used to be particularly common in Zaire. Early reports indicated limited neurological abnormalities in the cretins in this country, but one has to be cautious in interpreting these reports as comprehensive neurological examinations had not been performed (128). The movements are torpid and the reflex relaxation is usually much prolonged. However, hyperreflexia and Babinski signs were occasionally reported while knock knees and flat feet were obvious in the photographs of these patients in the literature. Subsequent expert neurological examination of some of these patients by De Long (120) suggested some of them had the neurological signs reported in the neurological type of cretinism, but they were partly obscured by the status of severe hypothyroidism. This is an important finding as it indicates in utero damage from hypothyroxinemia from maternal iodine deficiency does occur in myxedematous cretinism and is followed by severe, irreversible hypothyroidism in infancy and childhood.
Etiology and Pathophysiology of myxedematous cretinism
Three additional factors, acting alone or in combination, have been proposed for explaining the particularity of thyroid atrophy characteristic of the myxedematous type of cretinism (131):
1) Thiocyanate overload resulting from the chronic consumption of poorly detoxified cassava (88). Its role has been suggested in Zaire from the observation that populations in areas with severe but uniform iodine deficiency exhibit cretinism only when a critical threshold in the dietary supply of SCN is reached. SCN crosses the placenta and inhibits the trapping of iodide by the placenta and fetal thyroid (41, 90). This explanation is not necessarily relevant to other areas such as western China where myxedematous cretinism has been described.
2) Selenium deficiency. Severe selenium deficiency has been reported in Zaire in populations where myxedematous cretinism is endemic (95,98). Selenium is present in glutathione peroxidase (Gpx) that detoxifies H2O2 produced in excess in thyroid cells hyperstimulated by TSH because of iodine deficiency. Accumulation of H2O2 within the thyroid cells could induce thyroid cell destruction and thyroid fibrosis resulting in myxedematous cretinism. It has been proposed that the combination of deficiencies in iodine and selenium and SCN overload are required for the occurrence of severe thyroid failure during the perinatal period, and subsequent development of myxedematous cretinism (95).
3) Immunological mechanisms. Some authors (132,133) but not others (134) suggested immunological factors cause destruction of the thyroid, both in endemic and sporadic congenital hypothyroidism. The role of autoimmunity in the etiology endemic cretinism remains controversial.
ASSESSMENT OF IODINE STATUS IN POPULATIONS
Four methods are generally recommended for assessment of iodine nutrition in populations: urinary iodine concentration (UI), the goiter rate, serum thyroid stimulating hormone (TSH), and serum thyroglobulin (Tg) (see overview in Table 6). These indicators are complementary, in that UI is a sensitive indicator of recent iodine intake (days) and Tg shows an intermediate response (weeks to months), whereas changes in the goiter rate reflect long-term iodine nutrition (months to years).
Thyroid size
Two methods are available for measuring goiter: neck inspection and palpation, and thyroid ultrasonography. By palpation, a thyroid is considered goitrous when each lateral lobe has a volume greater than the terminal phalanx of the thumbs of the subject being examined. In the classification system of WHO (1), grade 0 is defined as a thyroid that is not palpable or visible, grade 1 is a goiter that is palpable but not visible when the neck is in the normal position (i.e., the thyroid is not visibly enlarged), and grade 2 goiter is a thyroid that is clearly visible when the neck is in a normal position (see Figure 6). Goiter surveys are usually done in school age children.
However, palpation of goiter in areas of mild iodine deficiency has poor sensitivity and specificity; in such areas, measurement of thyroid volume (Tvol) by ultrasound is preferable (135). Thyroid ultrasound is non-invasive, quickly done (2-3 mins per subject) and feasible even in remote areas using portable equipment. However, interpretation of Tvol data requires valid references from iodine-sufficient children. In a recent multicenter study, Tvol was measured in 6-12 y-old children (n=3529) living in areas of long-term iodine sufficiency on five continents. Age- and body surface area- specific 97th percentiles for Tvol were calculated for boys and girls (136). Goiter can be classified according to these international reference criteria, but they are only applicable if Tvol is determined by a standard method (1).
In areas of endemic goiter, although thyroid size predictably decreases in response to increases in iodine intake, thyroid size may not return to normal for months or years after correction of iodine deficiency (137,138). During this transition period, the goiter rate is difficult to interpret, because it reflects both a population’s history of iodine nutrition and its present status. Aghini-Lombardi et al. (138) suggested that enlarged thyroids in children who were iodine deficient during the first years of life may not regress completely after introduction of salt iodization. If true, this suggests that to achieve a goiter rate <5% in children may require that they grow up under conditions of iodine sufficiency. A sustained salt iodization program will decrease the goiter rate by ultrasound to <5% in school-age children and this indicates disappearance of iodine deficiency as a significant public health problem (1). WHO recommends the total goiter rate be used to define severity of iodine deficiency in populations using the following criteria: <5%, iodine sufficiency; 5.0%–19.9%, mild deficiency; 20.0%–29.9%, moderate deficiency; and >30%, severe deficiency (1).
Urinary iodine concentration (UIC)
Because >90% of ingested iodine is excreted in the urine, UI is an excellent indicator of recent iodine intake. UI can be expressed as a concentration (µg/L), in relationship to creatinine excretion (µg iodine/g creatinine), or as 24-hour excretion (µg/day), which is termed urinary iodine excretion (UIE). For populations, because it is impractical to collect 24-hour samples in field studies, UI can be measured in spot urine specimens from a representative sample of the target group, and expressed as the median, in µg/L (1). Variations in hydration among individuals generally even out in a large number of samples, so that the median UI in spot samples correlates well with that from 24-hour samples. For national, school-based surveys of iodine nutrition, the median UI from a representative sample of spot urine collections from ≈1200 children (30 sampling clusters x 40 children per cluster) can be used to classify a population’s iodine status (1) (Table 7).
However, the median UI is often misinterpreted. Individual iodine intakes, and, therefore, spot UI concentrations are highly variable from day-to-day (139), and a common mistake is to assume that all subjects with a spot UI <100 µg/L are iodine deficient. To estimate iodine intakes in individuals, 24-hour collections are preferable, but difficult to obtain. An alternative is to use the age-and sex adjusted iodine:creatinine ratio in adults, but this also has limitations (140). Creatinine may be unreliable for estimating daily iodine excretion from spot samples, especially in malnourished subjects where creatinine concentration is low. Daily iodine intake for population estimates can be extrapolated from UI, using estimates of mean 24-hour urine volume and assuming an average iodine bioavailability of 92% using the formula: Urinary iodine (µg/L) x 0.0235 x body weight (kg) = daily iodine intake (5). Using this formula, a median UI of 100 μg/L corresponds roughly to an average daily intake of 150 μg.
Thyroid stimulating hormone
Because serum thyroid stimulating hormone (TSH) concentration is determined mainly by the level of circulating thyroid hormone, which in turn reflects iodine intake, TSH can be used as an indicator of iodine nutrition. However, in older children and adults, although serum TSH may be slightly increased by iodine deficiency, values often remain within the normal range. TSH is therefore a relatively insensitive indicator of iodine nutrition in adults (1). In contrast, TSH is a sensitive indicator of iodine status in the newborn period (141, 142). Compared to the adult, the newborn thyroid contains less iodine but has higher rates of iodine turnover. Particularly when iodine supply is low, maintaining high iodine turnover requires increased TSH stimulation. Serum TSH concentrations are therefore increased in iodine deficient infants for the first few weeks of life, a condition termed transient newborn hypothyroidism or hyperthyrotropinemia. In areas of iodine deficiency, an increase in transient newborn hypothyroidism, indicated by >3 % of newborn TSH values above the threshold of 5 mIU/L in whole blood collected 3 to 4 days after birth, suggests but is not diagnostic of iodine deficiency in the population (141, 142). TSH is used in many countries for routine newborn screening to detect congenital hypothyroidism. If already in place, such screening offers a sensitive indicator of population iodine nutrition providing the timing of heel-stick blood collection is standardised and the TSH assay is modified to report TSH values in the range from normal to the cut-off level for diagnosis of congenital hypothyroidism (141). Newborn TSH is an important measure because it reflects iodine status during a period when the developing brain is particularly sensitive to iodine deficiency.
Thyroglobulin
Thyroglobulin (Tg) is synthesized only in the thyroid, and is the most abundant intrathyroidal protein. In iodine sufficiency, small amounts of Tg are secreted into the circulation, and serum Tg is normally <10 µg/L (143). In areas of endemic goiter, serum Tg increases due to greater thyroid cell mass and TSH stimulation. Serum Tg is well correlated with the severity of iodine deficiency as measured by UI (144). Intervention studies examining the potential of Tg as an indicator of response to iodized oil and potassium iodide have shown that Tg falls rapidly with iodine repletion, and that Tg is a more sensitive indicator of iodine repletion than TSH or T4 (145,146). However, commercially-available assays measure serum Tg, which requires venipuncture, centrifugation and frozen sample transport, which may be difficult in remote areas.
A new assay for Tg has been developed for dried blood spots taken by a finger prick (147,148), simplifying collection and transport. In prospective studies, dried blood spot Tg has been shown to be a sensitive measure of iodine status and reflects improved thyroid function within several months after iodine repletion (147,148). However, several questions need to be resolved before Tg can be widely adopted as an indicator of iodine status. One question is the need for concurrent measurement of anti-Tg antibodies to avoid potential underestimation of Tg; it is unclear how prevalent anti-Tg antibodies are in iodine deficiency, or whether they are precipitated by iodine prophylaxis (149). Another limitation is large interassay variability and poor reproducibility, even with the use of standardization (143). This has made it difficult to establish normal ranges and/or cutoffs to distinguish severity of iodine deficiency. However, recently international reference ranges for DBS Tg in iodine-sufficient school children (4-40 μg/L) has been made available (147) and a similar reference range proposed for DBS Tg in iodine sufficient pregnant women (150).
Thyroid hormone concentrations
In contrast, thyroid hormone concentrations are poor indicators of iodine status. In iodine-deficient populations, serum T3 increases or remains unchanged, and serum T4 usually decreases. However, these changes are often within the normal range, and the overlap with iodine-sufficient populations is large enough to make thyroid hormone levels an insensitive measure of iodine nutrition (1).
Table 6. Indicators of iodine status at population level
Indicator (units) |
Age Group | Advantages | Disadvantages | Application |
Median urinary iodine concentration (μg/L) |
School-age children, adults and pregnant women |
§ Spot urine samples are easy to obtain § Relatively low cost § External quality control program in place
|
§ Not useful for individual assessment § Assesses iodine intake only over the past few days § Meticulous laboratory practice needed to avoid contamination § Sufficiently large number of samples needed to allow for varying degrees of subject hydration |
See Table 7 |
Goiter rate by palpation (%) | School-age children |
§ Simple and rapid screening test: § Requires no specialized equipment |
§ Specificity and sensitivity are low due to a high inter-observer variation § Responds only slowly to changes in iodine intake |
Degree of IDD by goiter rate : § 0-4.9% - None § 5-19.9% - Mild § 20-29.9% - Moderate § ≥30% - Severe |
Goiter rate by ultrasound (%) | School-age children |
§ More precise than palpation § Reference values established as a function of age, sex, and body surface area |
§ Requires expensive equipment and electricity § Operator needs special training § Responds only slowly to changes in iodine intake |
|
Thyroid stimulating hormone (mIU/L) |
Newborns |
§ Measures thyroid function at a particularly vulnerable age § Minimal costs if a congenital hypothyroidism screening program is already in place § Collection by heel stick and storage on filter paper is simple |
§ Not useful if iodine antiseptics used during delivery § Requires a standardized, sensitive assay § Should be taken by heel-prick at least 48 hours after birth to avoid physiological newborn surge |
A <3% frequency of TSH values >5 mIU/L indicates iodine sufficiency in a population |
Serum or whole blood thyroglobulin (μg/L) |
School-age children and adults |
§ Collection by finger stick and storage on filter paper is simple § International reference range available § Measures improving thyroid function within several months after iodine repletion |
§ Expensive immunoassay § Standard reference material is available, but needs validation |
Reference interval in iodine-sufficient children is 4-40 μg/L
|
Table 7. Epidemiological criteria for assessing iodine nutrition in a population based on median and/or range of urinary iodine concentrations (from ref.1).
Median urinary iodine (μg/L) | Iodine intake | Iodine nutrition |
School-aged children | ||
<20 | Insufficient | Severe iodine deficiency |
20-49 | Insufficient | Moderate iodine deficiency |
50-99 | Insufficient | Mild iodine deficiency |
100-199 | Adequate | Optimal |
200-299 | More than adequate | Risk of iodine-induced hyperthyroidism in susceptible groups |
>300 | Excessive | Risk of adverse health consequences (iodine-induced hyperthyroidism, autoimmune thyroid disease and hypothyroidism) |
Pregnant women | ||
< 150 | Insufficient | |
150 – 249 | Adequate | |
250 – 499 | More than adequate | |
≥ 500 | Excessivea | |
Lactating womenb | ||
< 100 | Insufficient | |
≥ 100 | Adequate | |
Children less than 2 years old | ||
< 100 | Insufficient | |
≥ 100 | Adequate |
a The term “excessive” means in excess of the amount required to prevent and control iodine deficiency.
b In lactating women, the figures for median urinary iodine are lower than the iodine requirements because of the iodine excreted in breast milk.
IODINE FORTIFICATION AND SUPPLEMENTATION
Iodized salt
Iodized salt is considered the most appropriate measure for iodine fortification (1). The advantage of iodized salt is that it is used by nearly all sections of a community, irrespective of social and economic status. It is consumed as a condiment at roughly the same level throughout the year. Its production is often confined to a few centers so that fortification can occur on a large scale and with better controlled conditions. There are two forms of iodine which can be used to iodize salt: iodide and iodate, usually as the potassium salt. Iodate is less soluble and more stable than iodide and is therefore preferred for tropical moist conditions. When used, both are generally referred to as "iodized" salt.
The daily requirement of iodine is 150 µg per person for adults (1). The level of iodization of salt has to be sufficient to cover this requirement, considering potential losses from the point of production to the point of consumption, including the expected shelf life. It also should take into account the per capita salt consumption in an area. Although salt consumption in the range 10-15 g per day is common in developed countries, this is regarded as excessive because of a potential increased risk of hypertension. Therefore, intakes in the range of 3-6 g per day, or even less, are being recommended. This potential reduction in salt intakes should be taken into account when setting iodine levels in fortified salt. Iodized salt can also be used as a feed supplement for cattle and other livestock in iodine deficient areas. Allowing for these factors, the level of iodine as iodate currently recommended to provide 150 ug of iodine per day is in the range of 20-40 mg per kg salt (1).
The packaging of the iodized salt is very important. Jute bags have been used extensively but in humid conditions, the salt absorbs moisture. The iodate dissolves and will drip out of the bag if it is porous, and much of the iodine will be lost; up to 75% over a period of nine months. To avoid this, waterproofing is required, achieved by a polythene lining inside the jute bag or else a plastic bag. The additional cost of a plastic bag may be justified by reduced iodine losses and the potential resale value of the bags (151,152).
The use of iodized salt in the prevention of IDD has been reviewed (151). The control of the iodine concentration in salt at production level should be performed by using titration methods to provide quantitative data or, in the case of imported salt, by using reliable test kits to provide qualitative data at the point of entry. Consignments with suspect iodine levels should be rechecked by titration. National monitoring programs should: 1) periodically check salt iodine levels in retail shops and households using reliable test kits; 2) conduct occasionally goiter prevalence surveys; and 3) regularly measure urinary iodine. In order to determine the proportion of households using adequately iodized salt in a large geographic area, it is recommended to use cluster surveys at the provincial or national levels. It is also recommended to identify high risk communities where there are inadequate proportions of households using adequately iodized salt.
Salt iodization remains the most cost-effective way of delivering iodine and of improving cognition in iodine-deficient populations (153). Worldwide, the annual costs of salt iodization are estimated at 0.02-0.05 US$ per child covered, and the costs per child death averted are US$ 1000 and per DALY gained are US$34-36 (153). Looked at in another way, prior to widespread salt iodization, the annual potential losses attributable to iodine deficiency in the developing world have been estimated to be US$35.7 billion as compared with an estimated US$0.5 billion annual cost for salt iodization, i.e., a 70:1 benefit:cost ratio (154).
Iodine supplements
In areas of iodine deficiency where iodized salt is not available, iodine supplements are recommended by expert groups for women of reproductive age, pregnant women and lactating women (1,155). All pregnant women should consume approximately 250 µg iodine daily (1,155). To achieve a total of 250 µg iodine ingestion daily, strategies may need to be varied based on country of origin; in some countries, iodized oil supplements (see below) given once a year may be appropriate, in others, potassium iodide supplements may be given daily (1,155). In most regions, including the United States, women who are planning pregnancy or currently pregnant, should supplement their diet with a daily oral supplement that contains 150 µg of iodine in the form of potassium iodide (155). This is optimally started at least 3 months in advance of planned pregnancy. There is no need to initiate iodine supplementation in pregnant women who are being treated for hyperthyroidism or who are taking LT4 (155).
Iodized oil
Iodized oil ("Lipiodol®") was first used for the correction of iodine deficiency in Papua New Guinea (73). Buttfield and Hetzel (156) demonstrated the effectiveness of a single iodized oil injection (4 ml) in correcting iodine deficiency for a period of up to 4 1/2 years. Another trial in the Western Highlands of New Guinea demonstrated prevention of endemic cretinism and a reduction in fetal and neonatal deaths in the iodine treated group, if the iodized oil injection was given before pregnancy (126). Goiter in the treated population often resolved one to three months after the injection. However, the administration of iodized oil to adults with multinodular goiters in several other countries has not seen resolution or even diminution in size of their goiters.
Extensive additional studies on the use of iodized oil in the correction and prevention of IDD have been conducted in Latin America, Africa, Asia and Eastern Europe (157). The physiology and pharmacology of iodized oil in goiter prophylaxis has been extensively reviewed (158). Experience has confirmed the convenience of 200-400 mg the oral administration of iodized oil at yearly intervals through the primary health care system at the village level. In general, the effect of oral administration lasts half the time of the same dose given by injection (159-161). In regions of moderate-to-severe iodine deficiency without effective salt iodisation, lactating women who receive one dose of 400 mg iodine as oral iodised oil soon after delivery can provide adequate iodine to their infants through breastmilk for at least 6 months, enabling the infants to achieve euthyroidism (162). Recommendations from WHO-UNICEF-ICCIDD for oral iodized oil supplementation of women and children are shown in Table 8.
.
Table 8. Recommendations for iodine supplementation in pregnancy and infancy in areas where <90% of households are using iodized salt and the median UI is <100 µg/L in schoolchildren (from ref.1)
Women of child bearing age |
A single annual oral dose of 400 mg of iodine as iodized oil OR A daily oral dose of iodine as potassium iodide should be given so that the total iodine intake meets the RNI of 150 µg/d of iodine. |
Women who are pregnant or lactating |
A single annual oral dose of 400 mg of iodine as iodized oil OR A daily oral dose of iodine as potassium iodide should be given so that the total iodine intake meets the new RNI of 250 µg/d iodine.
|
Children aged 0-6 months |
A single oral dose of 100 mg of iodine as iodized oil OR A daily oral dose of iodine as potassium iodide should be given so that the total iodine intake meets the of 90 µg/d of iodine
|
Children aged 7-24 months old |
A single annual oral dose of 200 mg of iodine as iodized oil as soon as possible after reaching 7 months of age OR A daily oral dose of iodine as potassium iodide should be given so that the total iodine intake meets the RNI of 90 µg/d of iodine |
An iodized oil supplementation program is necessary when other methods have been found ineffective or are inapplicable. Iodized oil can be regarded as an emergency measure for the control of severe IDD until an effective iodized salt program can be introduced. The spectacular and rapid effects of iodized oil in reducing goiter can be important in demonstrating the benefits of iodization, which can lead to community demand for iodized salt. In general, iodized oil administration should be avoided over the age of 45 because of the possibility of precipitating hyperthyroidism in subjects with longstanding goiter (see further in section VI 3).
The possibility of linking up an iodized oil program with other preventative programs, such as the Child Immunization Program, has been discussed (163). Great progress has been made with child immunization programs in Africa and Asia. To this series of measures, oral iodized oil administration could readily be added to cover young children over the first 2-5 years of life. Women of reproductive age would require separate coverage through the primary health care system, especially the family planning health care system or in antenatal services at the same time as with tetanus toxoid.
Iodized bread
Iodized bread has been used effectively in the State of Tasmania in Australia (164). Successful use of iodized bread was also reported in Russia (165). Since 2009 by law in Australia and New Zealand, all salt used in baking of bread and similar edible products must be iodized salt. Early results from urinary iodine monitoring in Australia since implementation of this mandatory practice has seen correction of mild iodine deficiency in the population as a whole (6).
Iodized water
Water has some of the advantages of salt as a vehicle for iodine fortification. Both are daily necessities and thus their iodization will reach the most vulnerable groups – the poor and the isolated. Water fortified at a regular rate with iodine provides the thyroid with a steady daily ration, which is physiologically desirable (166).
Systems for iodization of drinking water can be classified as follows:
- Silicone elastomers releasing iodine. A commercial version of this approach is the “Rhodifuse” system of Rhône-Poulenc-Rorer-Doman (now Adventis). Silicone matrices containing 30 % sodium iodide are placed in polyethylene baskets. When the baskets are placed in wells, sodium iodide is released into the water according to the porosity and the surface/volume ratio. Initially successfully used in Mali (167), the system was then used in Burkina Fasso and the Central African Republic. Limitations to the system were climatic conditions, the cost of the diffuser and its maintenance. A similar device was manufactured locally in Malaysia (168).
- Iodide added to running water in pipes. A commercial example is the Hydroline system used in Sicily (169). The apparatus consisted of a canister filled with coarse crystals of iodine through which water was diverted from the line by a pressure differential. The system was highly efficient with a cost estimated at $ 0.04/person/day. A similar system used in the Sarawak region of Malaysia resulted in the reduction of goiter rate from 61% to 30% within 9 months together with improved thyroid function (170).
- Iodide added to run-off water. This technique was used by Cao at all (171) in Southern Xinjiang, China. It resulted in an increase of iodine intake by plants, possible benefits on rice production, increased iodine uptake by the thyroids of sheep and chickens, increased iodine in eggs and increased survival of newborn lambs.
- Manual addition of iodine to standing water. In this approach, iodine is added directly to vessels containing drinking water. It has been used most notably in northern Thailand, particularly to improve iodine nutrition in schoolchildren.
A review of water iodization programs (166) concluded that when properly monitored, the procedure is efficient in controlling iodine deficiency in smaller communities. But it is generally more expensive than iodized salt in large-scale national programs and that it is unlikely to be self-sustaining in poor rural countries and thus requires permanent external funding.
CURRENT GLOBAL STATUS OF IDD CONTROL PROGRAMS
Until 1990, only a few countries Switzerland, some of the Scandinavian countries, Australia, the U.S. and Canada were completely iodine sufficient. Since then, globally, the number of households using iodized salt has risen from <20% to >70%, dramatically reducing iodine deficiency (172). This effort has been achieved by a coalition of international organizations, including ICCIDD (now IGN), WHO, MI and UNICEF, working closely with national IDD control committees and the salt industry; this informal partnership was established after the World Summit for Children in 1990.
The two most commonly used approaches to assessing iodine nutrition on the population level are estimation of the household penetration of adequately iodized salt (HHIS) and measurement of urinary iodine concentrations (UICs) (173). UIC surveys are usually done in school aged children (SAC), because they are a convenient population, easy to reach through school based surveys and usually representative of the general population (173). Therefore, WHO use UICs from 6-12 y-old children in nationally-representative surveys, expressed as the median in µg/L, to classify a population’s iodine status (Table 1). More countries are beginning to carry out studies in high-risk population groups, i.e. women of reproductive age, pregnant women and younger children, however data is limited and the majority of countries still conduct routine iodine monitoring in SAC (174).
In 2017, representative UIC surveys are available for 139 countries. There are no up-to-date UIC data available for 55 countries. Available UIC data now cover >98% of the world’s population of SAC (174).
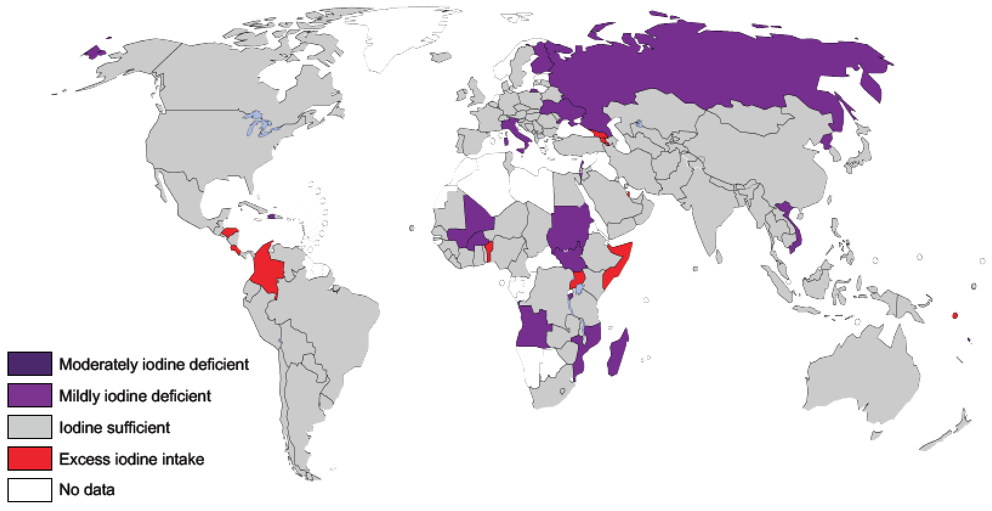
Figure 7: Shows countries classified by iodine nutrition in 2017 according to degree of public health importance based on the median UIC. Iodine intake is inadequate in 19 countries, adequate in 110 and excessive in 10. There are no up-to-date UIC data available for 55 countries. Reference (174).
Overall, approximately 75% of households worldwide have access to iodized salt. Those with the greatest access are living in the WHO regions of the Western Pacific and the Americas, and those with the least access are residing in the Eastern Mediterranean region (175,176).
The International Child Development Steering Group identified iodine deficiency as one of four key global risk factors for impaired child development where the need for intervention is urgent (177). But controlling IDD in the remaining 1/3rd of the global population at risk will not be easy. Although the key contributors to successful national programs have been identified (1), reaching economically disadvantaged groups living in remote areas and convincing small scale salt producers to iodize their salt are major challenges. An important strategy will be to strengthen national coalitions that include government partners, national and international agencies, the health-care sector and salt producers. In the countries that have begun iodized salt programs, sustainability will become a major focus. These programs are fragile and require a long-term commitment from governments. In several countries where iodine deficiency had been eliminated, salt iodization programs fell apart, and iodine deficiency recurred (178). Children in iodine deficient areas are vulnerable to even short-term lapses in iodized salt programs (179). To this end, countries should monitor the state of their iodine nutrition every three years and report to the World Health Assembly on their progress (180).
Advocacy should focus on damage to reproduction and cognitive development. Governments need to understand the serious impact of iodine deficiency; many still equate iodine deficiency with goiter, a mostly cosmetic problem and thus a low priority. IDD is one of the most important causes of preventable neurocognitive impairment worldwide, and elimination of IDD can contribute to at least five of the Millennium Development Goals (181): 1) Eradicate extreme poverty and hunger; 2) Achieve universal primary education; 3) Reduce child mortality; 4) Improve maternal health; and 5) Develop a global partnership for development. The World Bank (182) strongly recommends that governments invest in micronutrient programs, including salt iodization, to promote development, concluding: “Probably no other technology offers as large an opportunity to improve lives at such low cost and in such a short time.”
MONITORING THE IMPACT OF PROGRAMS OF SALT IODIZATION
The social process for successful implementation of a national IDD control program includes the following components (1):
- situation assessment
- communication of results to health professionals, political authorities and the public
- development of an action plan
- implementation of the plan
- evaluation of its impact at population level
The last phase, monitoring, is often neglected not only because it is the last phase in the process, but because it may be overshadowed by other components of the program such as implementation. In addition, many countries affected by IDD are low-income countries without the financial or technical resources to support a laboratory needed to properly monitor salt quality and iodine status.
The most cost-effective way to achieve the virtual elimination of IDD is through universal salt iodization, USI. The indicators used in monitoring and evaluating IDD control programs include both indicators to monitor and evaluate the salt iodization process, as well as indicators to monitor the impact of salt iodization on the target populations (these have been discussed previously).
Table 10 summarizes the criteria for monitoring progress towards sustainable elimination of IDD as a public health problem (1). It is considered that iodine deficiency has been eliminated from a country when:
- access to iodized salt at household level is at least 90%
- the median urinary iodine concentration is at least 100 μg/L and with less than 20 % of the samples below 50 μg/L
- when at least 8 of the 10 program indicators listed in Table 10 are implemented
Table 10. Summary of criteria for monitoring progress towards sustainable elimination of IDD as a public health problem (ref.1)
Indicators | Goals |
Salt Iodization Proportion of households using adequately iodized salt |
> 90 % |
Urinary iodine Proportion below 100 μg/L Proportion below 50 μg/L |
< 50 % < 20 % |
Programmatic indicators
|
Currently, there is much less information available on the impact of salt iodization programs than on the implementation of programs. The monitoring data of all countries affected by IDD are summarized country by country in an up-to-date database held by WHO (173).
Also, surprisingly, few longitudinal or case control studies have addressed the influence of USI on disorders induced by iodine deficiency, such as impairment of thyroid function, low birth weight, perinatal mortality and morbidity and the prevention of mental retardation. The oft-quoted statement that correction of iodine deficiency protects 50-100 million neonates from brain damage and mental retardation annually is politically attractive, but scientifically questionable. It results simply from a multiplication of the birth rate of the affected countries by the percentage of access to iodized salt at household level. Both figures lack precision. Moreover, this calculation implies that 100 % of neonates born in iodine deficient areas before the implementation of programs of iodine supplementation suffered intellectual impairment, which is a gross overestimation.
THE RISKS OF EXCESS IODINE INTAKE
As discussed so far in this chapter, iodine deficiency impairs thyroid function. Similarly, iodine excess, including overcorrection of a previous state of iodine deficiency, can also impair thyroid function. The effect of iodine on the thyroid gland is complex with a “U shaped” relation between iodine intake and risk of thyroid diseases. Both low and high iodine intake are associated with an increased risk of thyroid disorders. Healthy adults with normal thyroid glands can tolerate up to 600-1100 μg iodine/day without any side effects (5,183) (Table 11). However, this upper limit is much lower in a population which has been exposed to iodine deficiency for a prolonged period in the past. The optimal level of iodine intake to prevent any thyroid disease may be a relatively narrow range around the recommended daily intake of 150 μg (184).
Table 11. Tolerable upper intake level for iodine (µg/day)
Age group | EC/SCF, 2002 | IOM, 2001 |
1-3 years | 200 | 200 |
4-6 years | 250 | 300 |
7-10 years | 300 | 300 |
11-14 years | 450 | 300 |
15-17 years | 500 | 900 |
Adult | 600 | 1100 |
Pregnant women >19 years | 600 | 1100 |
Iodide goiter and iodine-induced hypothyroidism
When iodine intake is chronically high, as in coastal areas of Japan (91) due to daily intake of seaweeds rich in iodine or, in Eastern China, because of the high iodine content of the drinking water from shallow wells (92), the prevalence of thyroid enlargement and goiter is high, as compared to populations with normal iodine intakes. Also, the prevalence of subclinical hypothyroidism is elevated. The mechanisms behind this impairment of thyroid function are probably both iodine enhancement of thyroid autoimmunity and reversible inhibition of thyroid function by excess iodine (the Wolff-Chaikoff effect) in susceptible subjects (185). However, this type of thyroid failure has not been observed in neonates after the administration of huge doses of iodized oil to their mothers during pregnancy (162). Increased thyroid volume in children due to iodine excess has been observed when the median urinary iodine is >500 μg/L (186).
Iodine-induced hyperthyroidism, IIH
Iodine-induced hyperthyroidism (IIH) is the main complication of iodine prophylaxis. It has been reported in most iodine supplementation programs (187). But it is rare following a well executed program of iodine supplementation, for example as in Iran (188). The outbreak of IIH most extensively investigated occurred in Tasmania in the late 1960’s. This followed iodine supplementation simultaneously by tablets of iodide, iodized bread and the use of iodophors by the milk industry (189). The incidence of hyperthyroidism increased from 24/100000 in 1963 to 125/100000 in 1967. The disease occurred most frequently in individuals over 40 years of age with multinodular goiter and preexisting heart diseases (189). The most severe manifestations were cardiovascular and were occasionally fatal. The epidemic lasted about 10 to 12 years, but it was followed by an incidence of hyperthyroidism somewhat less than that existing prior to the epidemic.
The introduction of iodized salt in Zimbabwe resulted in a sharp increase in the incidence of IIH from 3/100000 to 7/100000 over 18 months (190). A high risk of IIH was also reported from Eastern Congo following the introduction of iodized salt (191). A multicenter study conducted in seven African countries, including Zimbabwe and Congo (192) showed that the occurrence of IIH in the last two countries was due to the sudden introduction of poorly monitored and excessively iodized salt in populations which had been severely iodine deficient for very long periods in the past. The conclusion of the study was that the risk of IIH was related to a rapid increment of iodine intake resulting in a state of acute iodine overload.
IIH following iodine fortification of salt cannot be entirely avoided even when fortification provides only physiological amounts of iodine. In a well-controlled longitudinal study in Switzerland, the incidence of hyperthyroidism transiently increased by 27% during one year after the iodine supply was increased from 90 μg/day to 150 μg/day (193).
The reason for the development of IIH after iodine fortification and/or supplementation is thought to be that iodine deficiency increases thyrocyte proliferation and mutation rates which, in turn, trigger the development of multifocal autonomous growth with scattered cell clones harboring activation mutations of the TSH receptors (194). Measurement of total intrathyroidal iodine by means of X-ray fluorescence scanning shows that only some nodules keep their capacity to store iodine, become autonomous and cause hyperthyroidism (195). It should be noted that there is considerable anecdotal evidence that increased iodine intake in patients with Graves’ disease may exacerbate hyperthyroidism in susceptible patients.
It thus appears that IIH can be considered one of the iodine deficiency disorders, and it may be largely unavoidable in the early phase of iodine repletion in iodine deficient populations, particularly in those with moderate to severe iodine deficiency. Its incidence reverts to normal or even below normal after one to ten years of iodine supplementation (196).
Iodine-induced thyroiditis
Another potential complication of excessive iodine intake is the aggravation or the induction of autoimmune thyroiditis by iodine supplementation. In experimental conditions, excessive iodine intake can precipitate spontaneous thyroiditis in genetically predisposed strains of beagles, rats or chickens (196). Potential mechanisms involved in iodine-induced thyroiditis in animal models include: 1) triggering of thyroid autoimmune reactivity by increasing the immunogenicity of thyroglobulin; and/or 2) damage to the thyroid and cell injury by free radicals.
Attention was drawn to iodine-induced thyroiditis in humans when studies conducted in the United States following the implementation of salt iodization showed an increased frequency of Hashimoto’s thyroiditis in goiters removed by surgery (197). Studies following the introduction of iodized oil in Greece pointed out the possible development of thyroid autoantibodies (198). Kahaly et al. (199) reported the development of thyroid autoantibodies and lymphocytic infiltration in 6 out of 31 patients with endemic goiter treated during 6 months with a dose of 500 μg potassium iodide (KI) per day. Acute massive iodine overload (daily consumption of at least 50 mg iodine daily) in healthy adults resulted in a sharp increase in thyroid peroxidase antibody titers together with elevated prevalence of goiter and serum TSH values. The prevalence of all abnormalities decreased after removal of iodine excess (202).
Finally, cross sectional studies of populations with different iodine intakes in Italy (63), Great Britain (64) and more recently in Denmark and Iceland (65) showed that the frequency of thyroid autoantibodies and hypothyroidism is higher in iodine replete populations than in iodine deficient populations. It is also recognized that the frequency of thyroid antibodies (200) and of autoimmune thyroiditis (201) is higher in the United States than in Europe, while the iodine intake is lower in Europe.
Hypothyroidism
To investigate the effects of iodine intake on thyroid disorders in China, a prospective 5-year survey was done in three rural communities with mildly deficient, more than adequate (previously mildly deficient iodine intake), and excessive iodine intake (median UIs of 88, 214 and 634 µg/L, respectively) (203,204). High iodine intakes did not increase rates of overt hypothyroidism or hyperthyroidism, but did increase cumulative incidence of subclinical hypothyroidism (0.2 percent, 2.6 percent, and 2.9 percent, respectively) and autoimmune thyroiditis (0.2 percent, 1.0 percent, and 1.3 percent, respectively). In most people, these disorders were not sustained.
Denmark has documented the pattern of thyroid disease after careful introduction of iodized salt. Pederson et al (205,206) prospectively identified new cases of overt hypothyroidism and hyperthyroidism in Denmark before and for the first 6-7 yr after introduction of iodized salt. There was a moderate increase in the incidence rate of overt hypothyroidism (RR = 1.35; 95% CI = 1.11-1.66) that occurred primarily in young and middle-aged subjects with previously moderate iodine deficiency. The overall incidence rate of hyperthyroidism also increased, from 102.8 to 138.7/100,000/year. But in contrast to IIH, many of the new cases were observed in younger adults (20-39 y), and were presumably of autoimmune origin.
Thyroid cancer (TC)
In animals, chronic overstimulation of the thyroid by TSH can produce thyroid neoplasms (207). However, the relationship between thyroid cancer and endemic goiter has long been debated without agreement on a possible causal relationship (208-212). The available evidence suggests iodine deficiency is a risk factor for thyroid cancer, particularly for follicular TC and possibly, for anaplastic TC (213). A recent review (213) concluded that: a) there are consistent data showing an increase in thyroid cancer (mainly follicular) in iodine deficient animals; b) there is a plausible mechanism (chronic TSH stimulation induced by iodine deficiency); c) there is consistent data from before and after studies of iodine prophylaxis showing a decrease in follicular thyroid cancer and anaplastic thyroid cancer; d) there is an indirect association between changes in iodine intake and thyroid cancer mortality in the decade from 2000 to 2010; e) autopsy studies of occult thyroid cancer show higher microcarcinoma rates with lower iodine intakes; and f) case control studies suggest a lower risk of TC with higher total iodine intakes.
It appears the prognosis of thyroid cancer is significantly improved following iodine supplementation due to a shift towards differentiated forms of thyroid cancer that are diagnosed at earlier stages. Overall, it appears that correction of iodine deficiency decreases the risk of, and the morbidity from, thyroid cancer (2013).
Thus, the benefits of correcting iodine deficiency far outweigh its risks (63, 214, 215). Iodine-induced hyperthyroidism and other adverse effects can be almost entirely avoided by adequate and sustained quality assurance and monitoring of iodine supplementation which should also confirm adequate iodine intake.
In summary, enormous progress has been made globally over the past two decades in understanding and eliminating iodine deficiency as the major cause of preventable brain damage in the fetus, newborn and infant and as a cause of thyroid disorders in adults. Three quarters of the world’s population now has access to iodine on a daily basis through edible iodized salt, but sustainability remains a challenge in many countries. Many recent excellent clinical research studies have revealed that IDD are not confined to remote, mountainous areas in developing countries as we once thought, but are a global public health problem that affects most countries, including developed countries and island nations (216). The recognition of the universality of iodine deficiency highlights the need for more research into the pathogenesis and consequences of mild to moderate iodine deficiency and the development of new strategies to establish and maintain sustainable IDD elimination.